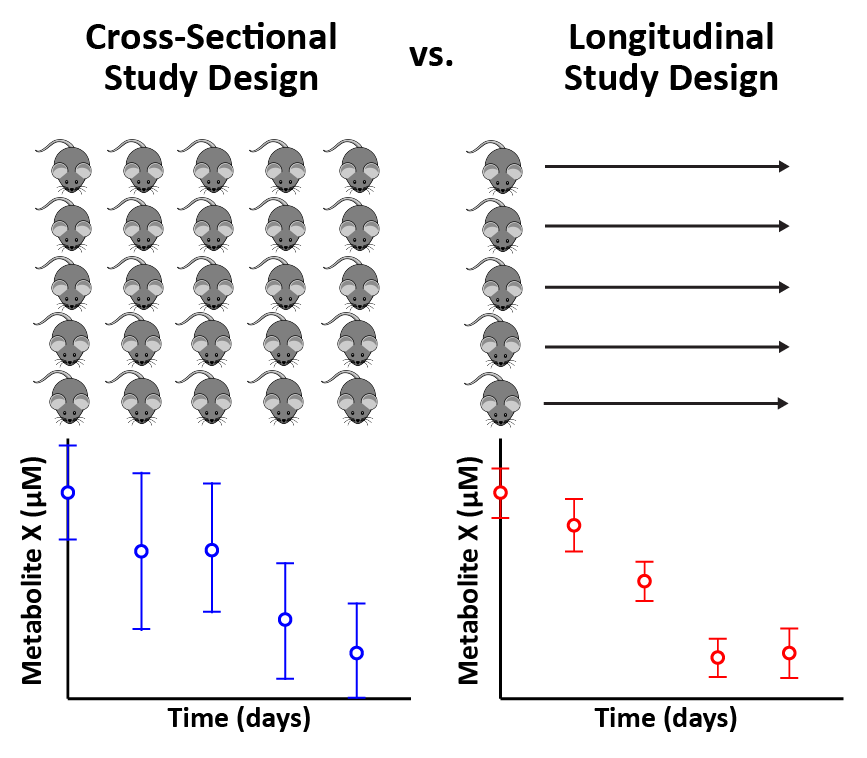
Welcome back to Article 8 in the 42 Factors Series of educational articles aimed at helping researchers improve data quality in omic experiments. In the previous article about choosing the Number of Replicates to use in metabolomic experiments, we noted that although biological variability is often large, the variability associated with time is often larger. Therefore, adding time points rather than statistical replicates usually yields a better return on investment in exploratory, range-finding studies. Today’s discussion will continue along that path with a discussion of cross-sectional vs. longitudinal study designs. The exciting conclusion promises to make everyone happy—from the biologists to data analysts and statisticians to institutional review boards and animal care and use committees. And we all just want to be happy, right?
Factor – Cross-Sectional vs. Longitudinal Study Design
Let me start by sharing why I care deeply about the subject of today’s article. The process of developing new treatments for disease (my lab focuses on cancer) requires evaluating an agent’s efficacy, safety/toxicity, pharmacokinetics/pharmacodynamics, mechanism of action, and potential biomarkers of response. Most agree that we should acquire as much data as possible from translationally relevant mammalian models prior to initiating human studies.
Early in my career, I noticed that many preclinical studies required sacrificing one animal to collect one data point. That inefficiency was due to the use of insensitive assay technologies. In a pivotal pharmacokinetic study in C57BL/6 mice during my graduate studies, I was fortunate to work with talented colleagues (Scott Hynes, Chris Landowski, Jianming Xiang, and John Hilfinger) who helped me derive clear conclusions from a minimal number of animals thanks to the use of mass spectrometry and a longitudinal study design. It was a pivotal moment that influenced the way I think about experimental design.
To my chagrin, years later I needed to conduct preclinical toxicity studies using clinical chemistry assays that required sacrificing one mouse to collect enough blood to conduct liver toxicity and renal toxicity assays—a cross-sectional study design. It occurred to me that perhaps thousands of laboratories around the world are still conducting clinical chemistry assays that require sacrificing unnecessarily large numbers of mice. I believe that needs to change. On behalf of both researchers and animals, I’m hoping to encourage efficient experimental designs coupled with sensitive assay technologies to deliver better data quality while minimizing the number of animals required.
Let’s get to it. There are a wide range of options when it comes to designing preclinical and clinical studies. We’ve already discussed that time course experiments are ideal for metabolomic studies and facilitate elucidation of cause-effect relationships, whereas stationary studies are less powerful.
Under the umbrella of time course experiments, two sub-types of study designs are cross-sectional and longitudinal. As illustrated in the cover image at the top of this article, a cross-sectional time course experiment involves merging and comparing data across different subjects at each time point. The primary disadvantages of cross-sectional designs are: 1) requirement for a greater number of subjects; 2) greater variability; and 3) inability to draw conclusions about cause and effect. Despite its limitations, a cross-sectional design must be invoked if the biological matrix of interest (e.g., tumor) can only be sampled once.
A longitudinal time course design, by contrast, involves comparing data within a single subject across multiple time points. The key advantages of longitudinal designs are that they: 1) require fewer subjects; 2) exhibit decreased variability (consequently, greater statistical significance); and 3) permit conclusions about cause and effect. Those advantages are notable; preclinical and clinical omic studies should be conducted longitudinally whenever possible.
As an example, a recent article examined the hypothesis that homocysteine levels are correlated with hypertension using both cross-sectional and longitudinal study designs. Only longitudinal analysis yielded the statistically significant conclusion that elevated homocysteine concentration is a predictive biomarker of hypertension. Cross-sectional data exhibited the same trend but were not statistically significant.
Still need more convincing about the power of longitudinal experiments? Our team recently conducted a longitudinal study of drug pharmacodynamics in mice. We were wondering why a particular drug fuels curative responses in humans but not in mice; in other words, could we facilitate alignment of mouse and human data to improve the translational relevance of our mouse model? Because the data are unpublished, the figure below is a cartoon summary illustrating the key finding that blood levels of the pharmacodynamic marker were elevated between treatments, suggesting that mice were not being cured due to sub-optimal treatment.
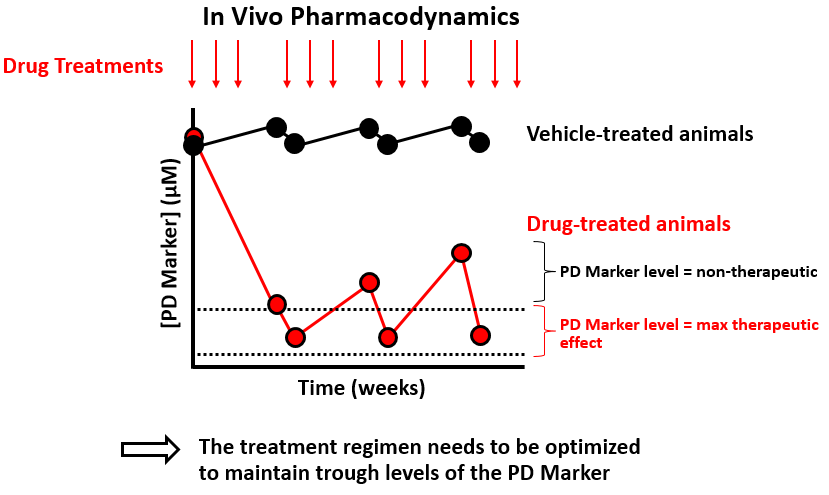
After optimizing the treatment regimen to maintain trough levels of the pharmacodynamic marker, mice exhibited curative responses (data not shown). Overall, this example demonstrates both the power of longitudinal study design and how metabolomics can be leveraged to develop translationally relevant mammalian models of disease and treatment.
Despite the advantages of longitudinal studies, one acceptable excuse for not conducting a longitudinal experiment is when the target matrix is an organ or tissue, which typically requires euthanasia immediately prior to sample collection.
We’ve spent the majority of this article discussing preclinical and clinical studies, but does longitudinal study design have relevance to cell-based experiments? Yes. I think it’s wise to design cell experiments through the lens of longitudinal studies. In particular, investigators should ensure that all samples across (e.g., vehicle- vs. drug-treated) and within (e.g., t=0 vs. t=2 h) series/groups are controlled so that only one variable is modulated. For example, by using techniques such as differential seeding and pre-normalization, one can minimize variation in cell number and confluence, which are factors that might affect metabolic endpoints. Thinking of a cell-based experiment as longitudinal isn’t technically accurate, since the subject (cells) is not constant across measurements, but the longitudinal construct is nevertheless useful to keep in mind while designing cell-based studies.
Take-home messages: 1) longitudinal study designs require fewer subjects; 2) longitudinal study designs exhibit decreased variability and, consequently, greater statistical significance; 3) longitudinal study designs are facilitated by technical advances in assay sensitivity; and 4) longitudinal designs require that the biological matrices being sampled (e.g., whole blood, plasma, serum, urine, saliva, stool) are accessible repeatedly without harming the subject.
Thanks to present and former members of my team for their efforts toward studying this factor, especially Wai Kin Chan, Leona Martin, Lin Tan, Eric Yin, and Tom Horvath.
Do you have any pressing metabolomics questions? Leave a comment.
0 comments